Earlier this week, one of our data analytics experts spoke at the Customer Behaviour Analytics conference in London. Rachit Khare was invited to cover the topic of driving innovation in consumer businesses – and he used his session to share how The Smart Cube addresses this challenge through agile experimentation. Below we’ve shared some key insights from Rachit’s presentation.
The consumer business landscape
The fundamental objectives for consumer businesses have remained the same over the years – drive growth, increase margins, provide great customer experience… and a few more. But while these fundamentals may not have changed, consumer businesses realise they now operate in a very different world certainly to that of ten, and actually even five years ago.
There is growing competition in most sectors as start-ups try to shake up the old guard. Most industries are facing huge pressures on margins and some are seeing stagnant or declining growth.
And while both these factors have always existed, the rapid emergence of new tech and the swathes of new data it produces, in combination present unprecedented challenges for traditional retailers.
Businesses are faced with an increasingly complex world; blockchain, AI, AR, VR, 5G – all massive disruptors. Some have already started impacting our world, and others are on the cusp of breaking out.
Driven by this, customer journeys and engagement with brands are changing, and so are their expectations. Surrounded by digital apps, wearable devices, and connected homes, consumers today are constantly connecting with brands and sharing their data. What they expect in return is a personalised and seamless experience, and armed with social media, they are quick to voice their opinions publicly, both good and bad, and to great effect.
If you are a brand operating in this environment, you likely will be both excited and nervous – perhaps in equal measures!
Contrast this to the operational reality in most businesses. Skill gaps, compounded by squeezed budgets to hire talent and drive change. Slow adoption of innovative technologies – driven both by budget constraints and bureaucratic roadblocks. Data accessibility and quality issues – which is an irony given the volume of data being generated, and availability of cheaper computation. And while the customer is looking for a unified experience, many businesses still have operational silos.
All of this leads to stifled innovation in businesses – quite the opposite of what’s needed in today’s world.
Introducing customer analytics
This is the operational playground in which customer analytics teams work.
While there are undoubtedly challenges, we believe there are also unique opportunities, if businesses approach this with the right mindset and toolkit.
So how can we convert some of these challenges into opportunities, and how can data science teams be the beacon of change?
The answer – “Agile Data-Driven Innovation”. A mechanism to efficiently experiment with available data to drive innovative solutions for the most pressing business questions.
- Agile – efficient and flexible
- Data-Driven – leveraging advanced data science
- Innovation – new business-focused solutions
The framework
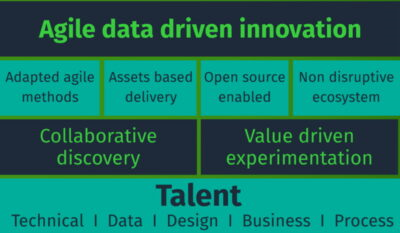
The framework to enable this starts with the right team – the foundation for any successful delivery. The ideal team includes not just the usual suspects – data scientists, technology and data owners – but also design experts, business stakeholders, end users, and change management leads. Collectively, this team is probably the single most important part of the puzzle.
The two cornerstones for successful deployment are – “collaborative discovery”, and “value-driven experiments”. Collaborative discovery focuses on identifying the right questions by engaging business and technical stakeholders. Closely linked, and as an outcome of this practice, is the principle of value-driven experimentation – where we put business value at the centre of selection, prioritisation, and design of solutions. Value needs to be measurable and auditable, because this really is the key to success.
In addition to these two, there are four principles which we believe are necessary:
- Adapted agile: to adapt principles of agile delivery from software development for analytics
- Assets-based delivery: a key enabler for efficiency so that teams don’t always have to start from scratch
- Open source: a lot of interesting algorithms are being made publicly available by leading tech firms, and we should adopt and customise those. No need to reinvent the wheel!
- Non-disruptive ecosystem: make sure you don’t break something till you have a better replacement!
Well it all sounds good in theory, but how does it look in practice? We will now talk about a real world case example, where we deployed a lot of these techniques…..
Driving innovation for a leading retailer
Over the last few years we have been working with one of the largest retailers in the UK.
The retailer has been on a transition journey, which began around a decade ago. The journey was focused on making the business truly data and analytics driven. To gradually move from BI focused on retrospective reporting, to using advanced data science at scale for business decisions.
A trigger for this change was the realisation of their own competitive vulnerability – they were at the risk of falling behind the market in understanding their customers, and in turn adapting their store and digital operations to the changing landscape. The business realised that the key was to truly put analytics and innovation at the centre of business decisions.
Getting started
But the task is easier said than done. Of course, there were gaps in data, tech, skills needed to support an analytics driven business, but the retailer had smart people, a strong BI team, a small advanced analytics team, and enough tech to get started. But there was still something missing – the mindset and framework to innovate using analytics.
Fundamental to this is to appreciate that innovation is not restricted to a purely technical domain, i.e., discovery and implementation of the latest in deep neural nets. It is as much about innovation in business processes and decisions, driven by better application of analytics.
Considering how analytics and business decisions feed into each other, it’s only natural they should be closely aligned within organisations, but this is very often not the case. It was true for our client as well. So the first big step in this journey was to find a senior champion for analytics – someone who understands quantitative modelling, but is also comfortable engaging with senior board members and driving change. This became the seed to grow analytics and conversations around analytics within the business. Further, this closer engagement with business enabled a collaborative discovery of the right questions – questions that will drive value if successfully delivered.
Working in an imperfect world
The next requirement was to adapt the business mindset – from seeking perfect project delivery conditions, to embracing imperfection. To be okay with current imperfections is not to deny the need for future improvements, but rather accepting incrementally better solutions because they provide continuous improvement. Of course, there would be some hard limitations which may make certain projects unattainable, but there are always opportunities lurking in the gaps – if we are willing to innovate.
As is often in most companies, our client had a range of partly connected databases, certain gaps in data quality and availability, and was operating on some traditional BI tools. All of this meant limited instances of complete data and limited/costly access to the right analytics toolkit. Our approach was to set up small agile teams focused on specific business questions, operating in a light deployment of permissible technologies, and with constant business engagement.
Focus on value
One of the challenges around implementing innovation using analytics is the misconception that it carries significantly higher risk than BAU. While innovation by its very nature will carry some risk, maybe even marginally more than tested BAU processes, the upside is significantly higher. There really is a trade-off between risk and reward, and each business will have its own agenda and parameters. In this case, considering its starting point, the retailer was focused on using analytics and innovation to drive more immediate business value, with greater blue-sky innovation reserved for a later stage.
Our approach to tackle this was to start with high priority business questions, “risk-bound” the experimentation by limiting scope, and focus on proving value from the proof-of-concept. Keeping the business closely engaged ensured we delivered MVPs in weeks back to users, who could then conceptually verify and even test in limited real-world scenarios. Only once we understood, estimated and agreed true business value, did the solutions go into the next phase.
Success breeds success
We got some big wins through this approach. For instance, we delivered a solution on one of the most common problems in consumer businesses – how to optimise Promotions Return on Investment. We successfully demonstrated business value of £4m from a PoC lasting 6-8 weeks, which scaled up to +£50m for the full solution.
There were similar success stories in other pockets of the business, including customer insights, store operations, and merchandising. All of this enabled the central analytics team to prove value in this new working model, and secure further business investments to improve data infrastructure and technologies. Further, it opened up the business to investing more in creating analytics assets, hiring more talent, and embracing advanced data science.
The long term view
Over the last couple of years we have been able to drive millions of pounds of benefit through application of agile analytics. More importantly, we have been able to do this cost effectively, resulting in significant return on investments on some of the flagship projects.
An equally significant change has happened in the way the business sees and uses analytics. While this is still an ongoing change, the business now approaches analytics to not only support BAU processes, but increasingly to drive innovation. An example of this is experimentation with the use of sensor technology in stores and advanced data science to understand shopper triggers to purchase.
At The Smart Cube we combine advanced analytics, data science and technology to solve our customers’ most pressing problems: from bespoke solutions such as merchandising analytics and revenue growth management, to comprehensive Analytics Centre of Excellence support.
If you’d like to learn more about how our analytics solutions could help your business adapt to changing consumer and market dynamics, please get in touch.