Every day brings exciting new AI and data science innovations – but which ones have true potential for your business? We sat down with Head of Analytics, Sudeepta Chaudhuri to find out how The Smart Cube’s new AI Lab is testing the business practicality of emerging AI approaches, and pioneering an ‘applied AI’ approach.
The pace of innovation in AI and data science has never been greater. Every day brings new frameworks, approaches, research and methodologies that promise to help us learn more, learn faster and gain greater value from our data than ever before.
Recently, The Smart Cube established its own AI Lab, to help make sense of these advances, and better understand how they can be practically applied by the kinds of organisations that work with us.
But what does the AI Lab actually do? How has it been designed? And most importantly, what will the lab’s work mean for the company’s current and future customers? We sat down with The Smart Cube’s Head of Analytics, Sudeepta Chaudhuri, to find out.
Q: Hi Sudeepta. Perhaps the best place to start would be with a short description of The Smart Cube’s AI Lab and what it does.
Sudeepta: Sure! The Smart Cube’s AI Lab is our new internal experimentation environment where we take known business challenges and test new AI algorithms and approaches in answering these questions.
So, as an example, we’d take a proposed AI use case – something like tag-based product matching using deep learning – and use open source models and tools, and third-party data sets, to see how well that works for data sets of varying sizes and complexities.
The goal is simple – to translate emerging AI approaches, models and technologies into proven, applied AI use cases.
Q: AI already plays a significant role in insight and intelligence delivery for some of your customers, so how does this differ from the work the team is already doing for them?
Sudeepta: In the AI Lab, our focus is on experimentation. We’ve developed an agile, sprint-based way of working that enables us to test lots of potential use cases and approaches very quickly.
We know that not all AI and data science models and approaches will be able to deliver value in all scenarios. The experiments we do here let us understand what’s really going to deliver value for the organisations we serve, in an environment where we can fail fast and move onto the next experiment without putting client projects at risk.
Q: Right now, new AI approaches and models seem to appear almost daily, so how does the lab decide which to focus on and investigate in-depth?
Sudeepta: Our AI Lab runs on principles of agile execution, where we onboard and implement new experiments in 3-4 week sprints. We draw in our candidate list of experiments by assessing the latest research in the field of ML with a high degree of practical applications, along with high-priority business challenges we uncover through our clients. After our initial assessment on the feasibility and potential business impact, we kick-off our experimentation sprint on the shortlisted topic.
Q: Can you share with us what the lab has been working on lately?
Sudeepta: At the moment, our broad focus is on deep learning – as there are a huge number of potential business applications in that area that are likely to be applicable for the kinds of organisations we work with frequently.
Within that, we’re looking at concepts of language, computer vision, text, speech, decision support – with the language being our first specific focus area. Our work has only just begun, and we’ve already seen great success in areas including deep learning sentiment classification, and product matching using NLP.
Q: What is the overarching vision for the lab, and what is its work going to mean for The Smart Cube and its customers going forward?
Sudeepta: The Smart Cube’s goal has always been to provide organisations with the highest quality intelligence and empower them to answer their most pressing and critical business questions. AI will undoubtedly play a huge role in that over the next decade and beyond.
The trouble is, there is no ‘one size fits all’ approach to AI. While many of the organisations we speak to regularly are hugely excited about what AI could mean for them and their operations, there are still far too many question marks around the how, where and when it can be applied successfully.
Our goal with the lab is to remove those question marks. We’re taking care of the experimentation, so we can provide organisations with viable AI support that we know can deliver results for their specific use cases.
Our focus is on applied AI. We’re taking the theoretical, and making it actionable, so our customers can embrace it with confidence and see real results from their AI investments.
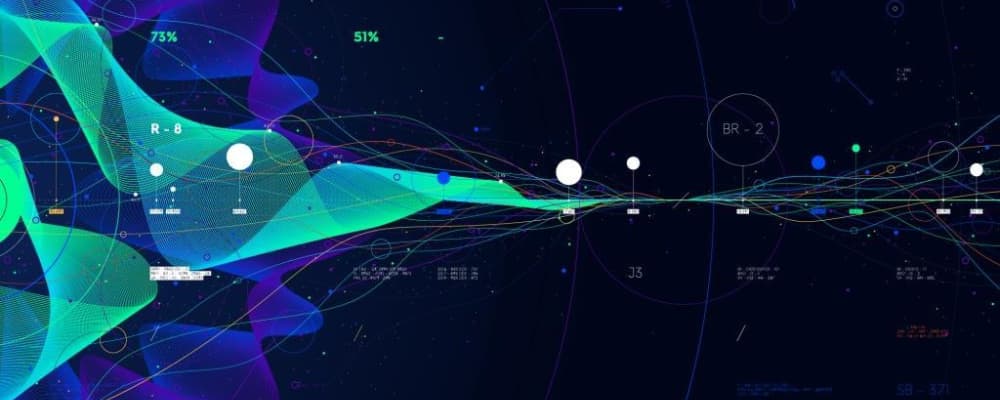
Q: Finally, where can people find out more about the lab, and learn how they can benefit from its applied AI experiments?
Sudeepta: The best place to start is over on the AI Lab page of our website. That’s where we’ll be sharing the results of our latest experiments, and giving organisations the opportunity to benefit from our unique experimentation approach.
Current AI LAB experiments
- Sentiment Classification using Deep Learning. This is a common NLP problem used for studying posts and reviews uploaded by users about a product, service, event or person. Through this experiment, we show the capability and strength of deep learning architectures and compare these model performances with lexical methods. Use include social media monitoring, customer feedback analysis and market research.
- Product Matching using NLP. Product matching is a specific application of the wider NLP task of text matching. This experiment is an application of novel deep learning architectures and transfer learning to match products based only on their titles and descriptions. This has applications in the retail and CPG industries, from price benchmarking to range rationalisation, and from competitor intelligence to recommendation engines.
- Transformers Language Interpretability. Transformer is a deep learning model used in NLP. Like recurrent neural networks, transformers are designed to handle sequential data, such as natural language, for tasks such as translation and text summarisation. However, unlike RNNs, they don’t require that the sequential data is processed in order. Here we interactively showcase transformer models and underlying concepts such as self-attention using Google’s Language Interpretability Tool (and others).
- Abstractive Text Summarisation using Transformers. Text summarisation is the process of automatically generating natural language summaries from an input document while retaining the important points. Here we use advanced NLP techniques to generate grammatically correct and insightful summaries for pharma research articles. As it helps in easy and fast retrieval of information, it can be used in financial research, summarisation of links (e.g. for automated newsletters) and market intelligence.